
A detailed application example related to the model predictive control of a water heater demonstrate that the proposed framework can be effectively used in modeling and control of dynamical systems. Thanks to the piecewise linear model structure the resulted regression tree can be easily utilized in model predictive control. Examples also demonstrate that the proposed model can effectively represent nonlinear dynamical systems. The basic idea behind a linear classifier two target classes can be separated by a hyperplane in the feature space. or a hyperplane, and this is what we use for multiple linear regression. Effectiveness of proposed model is demonstrated by benchmark regression datasets. Simple linear regression is when one independent variable is used to estimate a. In Ordinary Least Squares (OLS) Linear Regression, our goal is to find the line (or hyperplane) that minimizes the vertical offsets. Novel measures of model performance and complexity are developed to support the analysis and building of the proposed special model structure. The proposed method recursively identifies a hinging hyperplane model that contains two linear submodels by partitioning operating region of one local linear model resulting a binary regression tree. Random Forest Regression is a bagging technique in which multiple decision trees are run in parallel without interacting with each other. Random Forest Regression An effective Predictive Analysis.
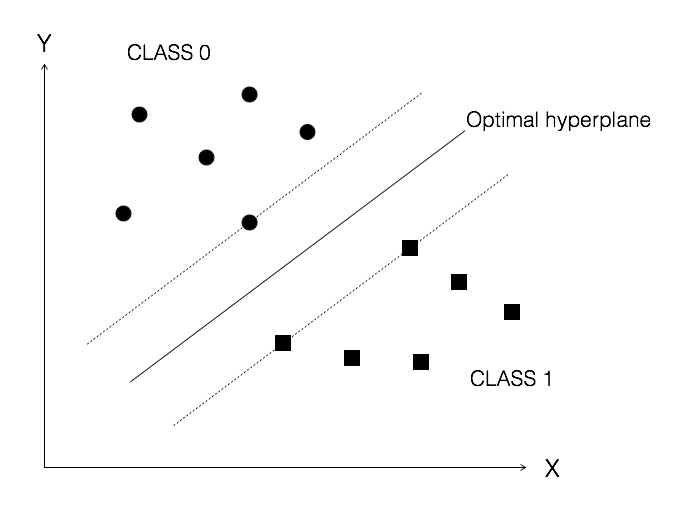
Hyperplan in linear regression how to#
In a special case ( c = 2), fuzzy c-regression clustering can be used for identification of hinging hyperplane models. This tutorial demonstrates a step-by-step on how to use the Sklearn Python Random Forest package to create a regression model.

A novel tool for regression tree identification is proposed based on the synergistic combination of fuzzy c-regression clustering and the concept of hierarchical modeling. conventional regression, but with weights that properly take account of the indi. Hierarchical fuzzy modeling techniques have great advantage since model accuracy and complexity can be easily controlled thanks to the transparent model structures. general a hyperplane of dimension one less than the space in which it is embedded.
